The clinical course of coronavirus disease 2019 (COVID-19) infection is highly variable with the vast majority recovering uneventfully but a small fraction progressing to severe disease and death. Appropriate and timely supportive care can reduce mortality and it is critical to evolve better patient risk stratification based on simple clinical data, so as to perform effective triage during strains on the healthcare infrastructure. This study presents risk stratification and mortality prediction models based on usual clinical data from 544 COVID-19 patients from New Delhi, India using machine learning methods. An XGboost classifier yielded the best performance on risk stratification (F1 score of 0.81). A logistic regression model yielded the best performance on mortality prediction (F1 score of 0.71).

P. K. Vinod, U. Deva Priyakumar
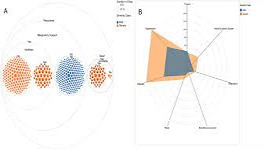